As a data analyst, you are in a unique position to harness the potential of ML to unlock valuable insights from data. ML algorithms enhance data analysis by efficiently processing vast data, revealing patterns and trends and enhancing decision-making.
However, mastering ML can be difficult. There is so much to learn and never enough time to learn them.
So, how do you set yourself up for success? Implement these 5 shifts in mindset.
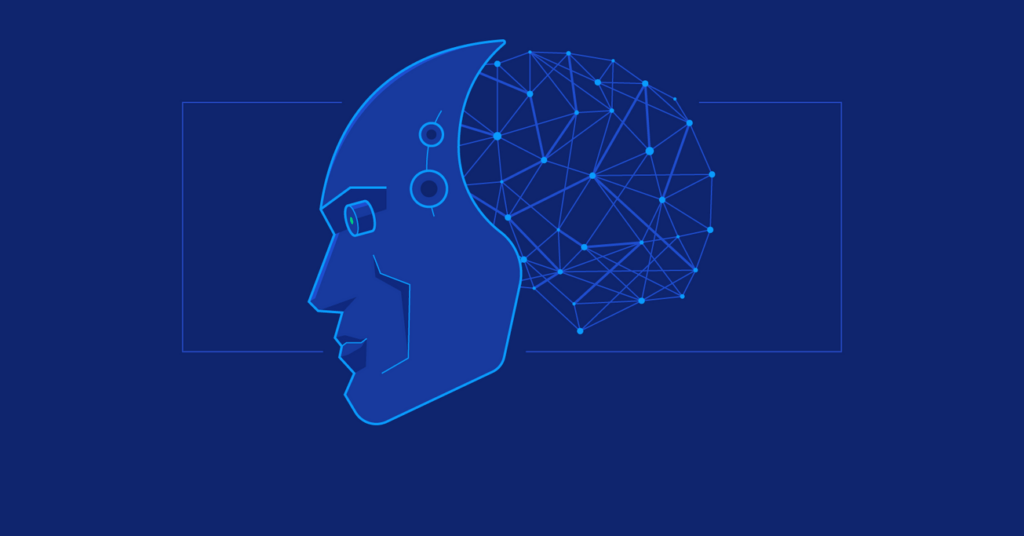
1. Believe in Yourself
– Acknowledge Your Expertise:
- Machine learning relies on large datasets, data manipulation, and exploratory analysis. A data analyst’s expertise in data manipulation and visualization allows them to preprocess and transform data for various algorithms. Their statistical analysis skills enable them to assess model performance and make data-driven decisions, paving the way for success in ML.
– Embrace Lifelong Learning:
- Recognize that learning is an ongoing journey. In the dynamic world of machine learning, keeping abreast of the latest techniques and tools is essential for your success. Staying informed about algorithm advancements, participating in workshops, conferences, and online forums, and embracing a lifelong learning attitude are vital for staying current and adapting to the ever-evolving ML landscape
– Celebrate Small Wins:
- Each successful data analysis you perform is a stepping stone towards mastering machine learning. Celebrate your achievements, even small ones, as every step forward brings you closer to becoming an expert in machine learning. This boosts confidence and motivation to continue learning and improving.
2. Overcome Challenges
– Start Small:
- Starting with simple machine learning projects improves your understanding of basic concepts and techniques. This will boost your confidence and motivate you to tackle more challenging tasks. As time goes on, each project you take on will deepen your knowledge of ML algorithms. After a while, your skill in designing and implementing advanced models will increase.
– Don’t be afraid to Fail:
- Failure is part of learning. Thus, you should treat it as an opportunity to learn and grow. Every unsuccessful attempt brings you closer to success. So, embracing failure allows you to understand your mistakes and identify where you can improve. When you understand what went wrong, you can adjust your approach and make better decisions in the future. So, see failure as a necessary and beneficial part of your journey towards success.
– Seek Support and Resources:
- Connecting with data scientists in online communities can provide valuable insights and encouragement. For example, when you join platforms like Kaggle or Stack Overflow, you may see new ideas and get help with your projects. Moreover, exploring online courses on platforms like Coursera or DataCamp can help you improve your ML skills.
3. Build Practical Skills
– Master Data Preprocessing:
- Clean, transform, and prepare your data effectively. A well-preprocessed dataset lays the foundation for a successful ML model. The data cleaning process involves removing duplicates, correcting values, and handling missing data. It also involves scaling, normalizing features, encoding variables, or reducing dimensionality.
– Understand Model Selection:
- Start with simpler models like linear regression or decision trees. As you gain experience and understanding of these simpler models, you will be better equipped to comprehend the intricacies of more complex algorithms. This gradual progression allows you to build a solid foundation and grasp the core principles of model selection. By starting with simpler models, you can also identify their limitations and know when it is necessary to employ more advanced techniques.
– Experiment and Iterate:
- Don’t be afraid to try different approaches. Experiment with different models, hyperparameters, and techniques to find what works best for your specific task. Exploring different methods can provide valuable insights and improve data understanding. Experimentation allows for refining models, hyperparameters, and techniques, ultimately leading to enhanced performance. There’s no one-size-fits-all solution, so think creatively and push the boundaries. Enjoy the learning curve for optimal results.
4. Leveraging Your Domain Knowledge
– Apply Domain Expertise:
- Your understanding of the industry or field you’re working in is invaluable. It allows you to frame business problems and interpret machine learning results effectively. Understanding the industry helps identify data sources, analyze features, identify biases, and collaborate with experts to ensure machine learning solutions align with business needs and goals, ensuring accuracy and reliability.
– Collaborate Across Disciplines:
- Engage with experts from different domains. Their perspectives can provide fresh insights and lead to more effective solutions. By engaging with experts from different domains, individuals can benefit from a diverse range of experiences and knowledge. These experts can offer unique perspectives that challenge conventional thinking and encourage innovative problem-solving approaches. By tapping into their expertise, individuals can enhance their understanding of complex issues and ultimately arrive at more effective and well-rounded solutions.
5. Focus on Making an Impact
– Focus on Business Value:
- Align your ML efforts with the strategic goals of your organization. Demonstrating tangible business impact is a powerful motivator. Aligning ML efforts with organizational strategic goals prioritizes and focuses efforts, ensuring a direct impact on goals. Successful ML initiatives demonstrate value, motivate innovation, and drive positive organisational change.
– Communicate Results Effectively:
- Translate complex machine learning concepts into clear, actionable insights that stakeholders can understand and use. By using simplified language and visual representations, ML concepts can be effectively communicated to stakeholders. This enables them to comprehend and apply these insights in making informed decisions. This translation process bridges the gap between technical jargon and practical applications, empowering stakeholders to leverage the power of ML in their respective domains.
Conclusion
Embracing ML as a data analyst opens up a world of possibilities. It’s not just about building models; it’s about using data to drive meaningful change and create value. Remember, every step you take, no matter how small brings you closer to achieving your goals. With determination, a willingness to learn, and a belief in your potential, you have the power to revolutionize how your organization leverages data. Embrace this journey with confidence, and let the world of machine learning unfold before you.